Predicting Wind Speed and Direction Using Machine Learning and Darts Framework


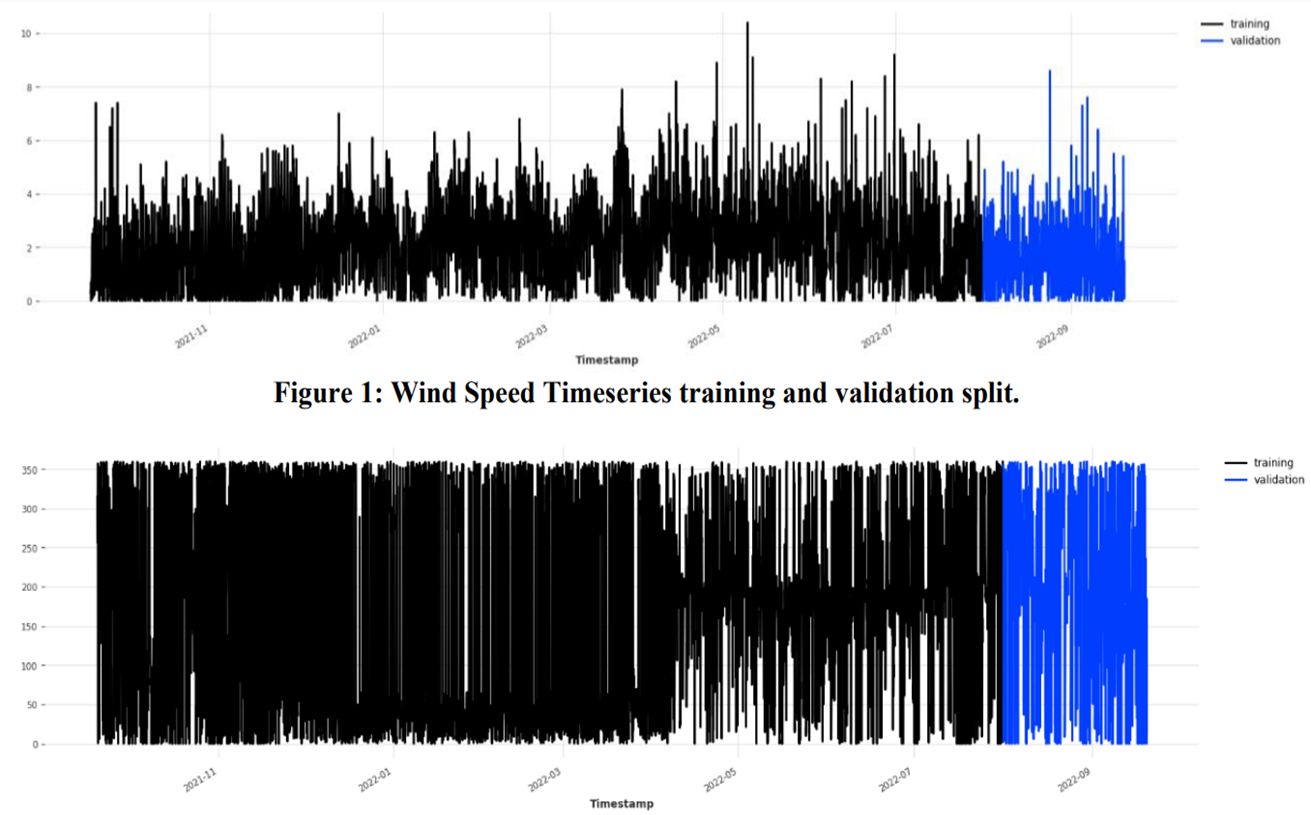
Description
UEL
August 25, 2023
View Publication here
Wind Speed and Direction Prediction Using Machine Learning Models in Darts Framework
This project aimed to enhance the accuracy and efficiency of wind speed and direction forecasting in Bauchi State, Nigeria, leveraging the Darts time-series framework. I evaluated ten machine learning algorithms across various forecast horizons (1 to 48 hours) using solar radiation and meteorological data. By incorporating covariates such as gust, global horizontal irradiance (GHI), and relative humidity, the models' predictive performance significantly improved.
Key contributions include:
- Conducting a comprehensive comparison of algorithms, with CatBoost achieving the best results for wind speed (RMSE: 0.877 at 12-hour lag) and wind direction (RMSE: 93.8 at 2-hour lag).
- Demonstrating that classical models outperform neural network models in accuracy and computational efficiency.
- Showcasing the value of Darts for improved computational efficiency and comparable or superior results to prior studies.
This work provides actionable insights for optimizing renewable energy planning and decision-making in Nigeria.